Abstract
The widespread use of artificial intelligence (AI) technologies has been a double-edged sword. On one hand, it has facilitated significant technological advances in the field of chemistry, greatly improving productivity; on the other hand, the readily available generative AI tools have intensified publication and academic integrity issues like never before. This article aims to give a brief introduction to the recent application of artificial intelligence (AI) in chemistry, and an overview of the integrity problems fuelled by generative AI tools.
A brief introduction to artificial intelligence
The 2024 Nobel Prizes for both chemistry and physics were awarded to people in the field of artificial intelligence (AI); the chemistry laureates for their development of AI tools that can accurately predict protein structure from sequence and vice versa and the physics laureates for their foundation work in machine learning.1 This demonstrates just how deeply AI has become integrated into science and our everyday life.
For people who are not quite so technology-savvy, AI has been something that has more recently entered our lives in the last few years. However, the first article on this topic was published in 1943 when McCulloch and Pitts reported a binary learning model that mimics human neurons.2 The term “artificial intelligence” was later coined in 1956 at a Dartmouth workshop.3 The mission of AI was defined as machine learning, where a machine is used to simulate intelligence by gathering and analysing data. However, limited by available computational power, this technology did not flourish until the 90s, when there were significant advances in data storage and graphic processing and large amounts of data became available through the internet.4 This development enabled the era of deep learning, which has the ability to perform complex processing from raw input, and subsequently led to generative AI that can create computer-synthesised content.4-5
AI can be trained with data to identify relationships, patterns and trends as well as make predictions or improvements based on the learnt information. These developments have led to significant changes in our life, education and research. Applications in chemistry include using this powerful information processing ability to analyse data, perform complex calculations, predict properties and activities of molecules, and to optimise methods and elucidate structures.6-7 Chemical Abstracts Service (CAS) Content Collection conducted an analysis of chemical publications between 2000 and 2020 to better understand the trend of AI and machine learning-related development.8 The number of AI-related chemical publications had a substantial increase post-2017, with the percentage of AI-related journal publications increasing from 0.2% to 1.2% from 2017 to 2020. These publications cover diverse fields in chemistry and are led by analytical chemistry, industrial chemistry and biochemistry. In response to the rapid growth, a biannual journal, Artificial Intelligence Chemistry, was established in 2023.9
This article aims to provide a brief introduction to the recent application of AI in chemistry, and an overview of the integrity problems fuelled by generative AI tools. The timeline in Fig. 1 summarises some of the milestones in the history of chemoinformatics and robotics that are covered in this article.
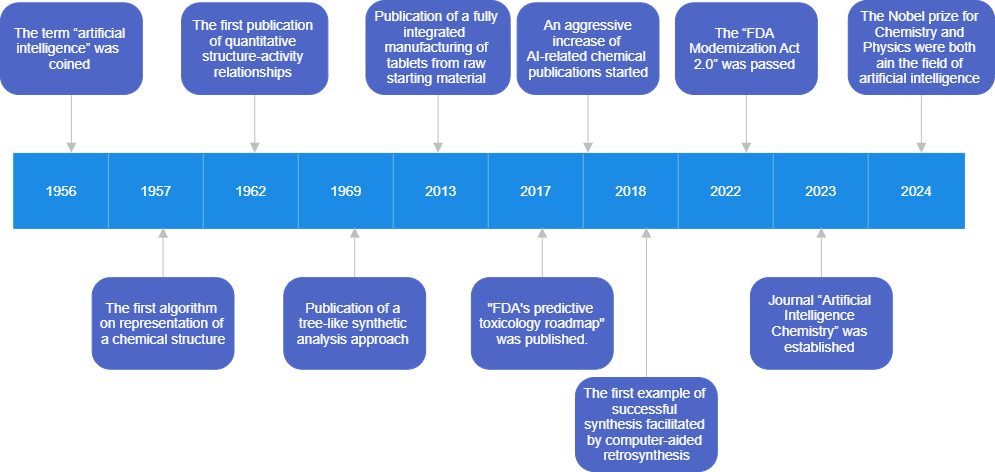
Chemoinformatics and recent AI-development
Computers can process vast amounts of data in a way that is impossible for humans. Chemistry is one of the first disciplines to adapt to computer science. The first algorithm was published by Ray and Kirsch in 1957 on 2D representation of a chemical structure. This representation is generally regarded as the start of chemoinformatics or chemical information science.10-11 However, the term “chemoinformatics” was not used until 1998, by Frank K. Brown in a discussion of information technology relating to drug discovery.12 Drug discovery has remained a particularly active area in AI-related development today.13
There are two primary areas of focus in chemoinformatics: molecular representation and property prediction. Unique, unambiguous and machine-readable representation of molecules is the basis of developing chemical information technologies and is still a critical issue for AI-related development today.14 After Ray and Kirsch’s publication, another algorithm on 2D presentation of chemical structure was reported in 1965 by H. L Morgan, and this representation system became the basis of the Chemistry Abstract Service (CAS) registry.15-16
The initial development of structural representation fostered an interest in computer-aided synthesis planning (CASP), signaled by Corey and Wipke’s description of a tree-like synthetic analysis approach in 1969,17 and this structure remains the basis for CASP today. One barrier to CASP is data scarcity. Although a vast number of reactions have been published, the majority of information is hidden behind subscription-based sources. A lot of the exploratory work was limited to an open-source database that was extracted only from United States’ patents, in which many reactions miss important information such as yield, reagents and conditions.18 The rise of open-source journals is improving the availability of reaction information. However, another problem with reaction data is the lack of information about failed reactions. Although it does not make sense to report your 100 failed reactions along with the one that finally worked, this information would be useful for AI models. Early work on CASP centred on developing representation of chemical reactions, but recent development in deep learning has shifted the focus to the extraction of reaction data from structural representations.19-21 In 2018, the first example of successful synthesis facilitated by computer-aided retrosynthesis was reported by Grzybowski’s group.22 Synthetic routes of the eight targets were tested in the lab and produced better results than the previous literature routes.23 Since then, a number of commercial retrosynthesis tools have been made available, such as those built on SciFinder, Reaxys and SynFin platforms,24-26 as well as open-source synthetic planning tools.27-28
The search for improved structural representation continues, and AI has offered new direction in this area. Simplified Molecular Input Line Entry System (SMILES) and International Chemical Identifier (InCHl) are commonly used machine-readable formats today.14 One example of using AI to improve structural representation was reported by Clevert et al.29 They used unsupervised leaning to “translate” SMILES representation to a new format and showed that the new format performs better for a virtual screen. Another example adopted an edited SMILES format for improved retrosynthetic planning.30
Pioneering work on property prediction was done on quantitative structure-activity relationships (QSAR), which used computational modelling to predict biological activity from chemical structure.31 The first work was reported by Hansch in 1962.32 Although only 20 phenoxyacetic acids were studied, Hansch was able to establish a mathematical model for the ability of these chemicals to promote plant growth based on partition coefficients. The recent development in QSAR has fueled a paradigm shift, especially in areas of toxicology33 and drug discovery13.
The Food and Drug Administration (FDA) in the United States has been a leader in modernising toxicology. A roadmap published in 2017 showed the FDA’s commitment to predictive toxicology.34 The recent development in Generative AI has led to advancement of multiple toxicology tools.35 For example, AnimalGAN allows testing of new compounds on virtual animal models, moving away from animal testing.36 TranslAI can “translate” experimental data from one domain to another, such as between different organs,37 male and female38 and different age groups.38 Furthermore, the “FDA Modernization Act 2.0” was passed in December 2022, which established the use of in silico modelling and simulation within the regulatory framework.39
In drug discovery, AI technology has revolutionised the pharmaceutical industry. AI tools have been applied in all stages of drug discovery including disease identification, target identification, screening, lead discovery, and clinical trials.13 The use of these technologies can significantly reduce the time and cost requirements associated with new drug development and drug repurposing.40-43 In particular, research for rare diseases with very low occurrence is hindered due to the lack of data obtainable from conventional methods.44-45 Technologies such as virtual screening, which are not limited by existing chemical libraries or structure-activity relationships (SAR) data, have enabled new developments using synthesised data.41 The first drug fully discovered and developed using AI in under 2 years entered clinical trial in 2024.46-47 Known as FB1006, this drug was developed to treat the orphan disease amyotrophic lateral sclerosis (ALS), which causes gradual loss of muscle movement. This event signaled a new era in drug discovery.
Automation and recent AI-development
Through software development, AI has accelerated automation for chemistry. Early automated synthesis machines had very limited capability and focused on removing repetitive tasks.48 The resulting products, such as peptide, oligosaccharide, and oligonucleotide synthesisers have been widely applied in scientific research. The search for a general synthesis machine has continued, leading to more technologies in areas such as on-demand synthesis, real-time monitoring and optimisation of reactions.6 AI-based development of smart control allowed real-time data collection to aid machine-powered optimisation and interfaces that can control and process data for multiple sensors, with these developments being made more affordable.49 Smart control has found application in many technologies to improve performance and increase the level of automation, notably in flow chemistry50-51 and high throughput screening.52-55
Continuous manufacturing based on flow chemistry has been serving chemical production since the end of 20th century, improving safety, enhancing production efficiency, reducing waste and making scaling up easier.56 Recent progress towards autonomous end-to-end continuous manufacturing has gained remarkable ground. An integrated system reported by Trout et al. has achieved pilot-scale manufacturing of tablets from raw starting material through a four-step synthesis.57
High throughput screening (HTS) has been widely used in biology, but the diverse reagents and reaction conditions limited application in chemistry. Developments in hardware and control interfaces have made significant advancements for its applications in chemistry and HTS has found applications in reaction optimisation, QSAR and materials science.52 A recently published example demonstrated the use of automated high-throughput experimental arrays to optimise reaction conditions, generate compound libraries and structure activity screening.55 In this work, a 1536-well plate was used to screen 80 amines under 8 conditions, and the products were analysed by LC-MS and in biological assays on 384-well plates. A high level of automation was demonstrated in the experiment design, execution, monitoring and result reporting in this study.
With the development of both software and hardware, the concept of self-driving labs has become reality, where AI-driven synthetic planning was coupled with automated equipment to plan and perform experiments with minimal human input.58-60 Applications of self-driving labs have been reported in molecule discovery,61 materials science62 and synthetic biology.63-64
Integrity issues with artificial intelligence
The emergence of AI-based technologies have served to improve productivity and efficiency in science and has even allowed us access to information unobtainable from conventional methods. However, the readily accessible nature of generative AI tools has intensified challenges regarding publication and education integrity.
Integrity issues such as plagiarism, fraudulent data and image manipulation have always existed in scientific publication. However, generative AI tools such as chatbots, image generators and data generators have made it much easier to fabricate articles. Large-scale production of fake research publications by companies known as “paper mills” have become a great problem.65-67 A computer analysis found that 1.7% of published articles had close textual similarity to those produced by paper-mills.66 In response to this problem, Technical & Medical Publishers (STM) integrity hub launched in 2021; it has 135 members and supports major publishers.68
Images in publishing have an even bigger problem. Proofig, an AI-tool to ensure image integrity launched in 2022, found problems in 25% manuscripts that it scanned.69 The integrity issue in publication has since spread to other areas with reports of “peer review mills” and “citation mills” where companies profit from fake peer reviews and selling citations.67,70
Publishers have started tackling these problems, resulting in the unprecedented retraction of over 10,000 papers in 2023, mostly with Chinese co-authors.71 This triggered a nationwide audit of research misconduct led by the Chinese government. On 6 January 2025, the Supreme Court in China released guidelines on protecting innovation and listed research fraud and publication misconduct in key areas to focus on.72
Academic integrity
Academic integrity in education has also faced significant challenges with the advent of generative AI technologies. Although AI can make education more accessible and efficient, there are some issues around how it is used by students and how educators can ensure their assessments remain adequate evidence of student learning. Tools such as AI-driven text generators, paraphrasers, and translation applications have made it easier for students to bypass traditional learning and assessment processes. Instances of students submitting AI-generated essays or code as their own work are increasingly reported, which complicates the task of educators and institutions to ensure authenticity in assessments.73
In New Zealand, NZQA have started implementing new policies to address these concerns, including updated guidelines for assessment moderation and AI detection tools.74 It is not clear whether AI has led to an increase in academic integrity issues or simply changed the tools available,75-76 but as it is here to stay, educators are having to adapt their courses and assessments to encourage students to avoid inappropriate use of AI and engage responsibly with AI resources. Some institutes, such as the University of Waterloo in Canada, have developed comprehensive guides for many types of assessment for how lecturers and teachers can adapt their assessments.77 Education literature also provides some recommendations, including lecturers and teachers openly citing how they’ve used AI in their material to set an example, incorporating discussions and activities around awareness of AI limitations, bias and inaccuracy, and having clear institutional policies around AI use.78
Final remarks
Despite all the exciting developments, wide application of AI-based technology still faces many challenges, such as data scarcity, low adoption rate, high cost and limited capacity of automated systems.79 However, the AI revolution has only just started. With increased investment and attention in this area, technologies are evolving at an unprecedented rate. While many are excited about the foreseen future of superintelligence,80 its full-scale impact on the economy, society and the human race must be carefully considered. As the 2024 Nobel Prize laureate, Geoffrey Hinton, pointed out during his banquet speech, AI has already been used to make people indignant, facilitate massive surveillance and cyber-crime, and if only concentrating on short-term profit, it could lead to catastrophic consequences.81 The Pandora’s box is already opened; we need to urgently improve our education and regulation systems to stay in control of the technology.82 Global collaboration is needed for this fight, all humans are in it together.
References
- Nobel Prize Outreach AB 2025. All Nobel Prizes 2024 https://www.nobelprize.org/all-nobel-prizes-2024/.
- McCulloch, W. S.; Pitts, W. H. The Bulletin of Mathematical Biophysics 1943, 5, 115–133.
- 3. McCarthy, J.; Minsky, M. L.; Rochester, N.; Shannon, C. E. AI Magazine 1955, 27, 12–12.
- 4. Muthukrishnan, N.; Maleki, F.; Ovens, K.; Reinhold, C.; Forghani, B.; Forghani, R. Neuroimaging Clinics of North America 2020, 30, 393–399.
- 5. LeCun, Y.; Bengio, Y.; Hinton, G. Nature 2015, 521, 436–444.
- 6. Ananikov, V. P. Artificial Intelligence Chemistry 2024, 2, 100075–100075.
- 7. Yang, L.; Guo, Q.; Zhang, L. Chemical communications 2024, 60, 6977–6987.
- 8. Baum, Z. J.; Yu, X.; Ayala, P. Y.; Zhao, Y.; Watkins, S. P.; Zhou, Q. Journal of Chemical Information and Modeling 2021, 61, 3197–3212.
- 9. Jiang, J.; Wang, S.; Mukamel, S. Artificial Intelligence Chemistry 2023, 1, 100001.
- Willett, P. Wiley Interdisciplinary Reviews: Computational Molecular Science 2011, 1, 46–56.
- Ray, L. C.; Kirsch, R. A. Science 1957, 126, 814–819.
- Brown, F. K. Chemoinformatics: What Is It and How Does It Impact Drug Discovery. In Annual Reports in Medicinal Chemistry, issue 33; Academic Press, 1998.
- Pushkaran , A. C.; Arabi, A. A. Artificial intelligence review 2024, 57.
- Polanski, J.; Gasteiger, J. Computer Representation of Chemical Compounds. In Handbook of Computational Chemistry; Leszczynski, J., Ed.; Springer, 2017; pp. 1997–2039.
- Morgan, H. L. Journal of Chemical Documentation 1965, 5, 107–113.
- Wills, T. J.; Polshakov, D. A.; Robinson, M. C.; Lee, A. A. Journal of Chemical Information and Modeling 2020, 60, 4449–4456.
- Corey, E. J.; W. Todd Wipke. Science 1969, 166, 178–192.
- Coley, C. W.; Green, W. H.; Jensen, K. F. Accounts of Chemical Research 2018, 51, 1281–1289.
- Coley, C. W.; Green, W. H.; Jensen, K. F. Accounts of Chemical Research 2018, 51, 1281–1289.
- Kearnes, S.; McCloskey, K.; Berndl, M.; Pande, V.; Riley, P. Journal of Computer-Aided Molecular Design 2016, 30, 595–608.
- Han, Y.; Deng, M.; Liu, K.; Chen, J.; Wang, Y.; Xu, Y.-N.; Dian, L. Chemistry - A European Journal 2024, 30.
- Klucznik, T.; Mikulak-Klucznik, B.; McCormack, M. P.; Lima, H.; Szymkuć, S.; Bhowmick, M.; Molga, K.; Zhou, Y.; Rickershauser, L.; Gajewska, E. P.; Toutchkine, A.; Dittwald, P.; Startek, M. P.; Kirkovits, G. J.; Roszak, R.; Adamski, A.; Sieredzińska, B.; Mrksich, M.; Trice, S. L. J.; Grzybowski, B. A. Chem 2018, 4, 522–532.
- Davey, S. G. Nature Reviews Chemistry 2018, 2, 0152.
- CAS. Retrosynthetic Analysis Software | CAS https://www.cas.org/solutions/cas-scifinder-discovery-platform/retrosynthetic-analysis-software?utm_campaign=APC_GEN_ANY_SCI_LDG &utm_medium=SCH_SUP_PAD&utm_source=Google&utm_content=&gad_source=1&gclid=CjwKCAiAp4O8BhAkEiwAqv2UqH51-vJ5a7PJyZNThBWqZ5SvPsuObOrZE-qmIl0akk2z3mbTPKY9LRo C2roQAvD_BwE (accessed 10/01/2025).
- Elsevier. Reaxys Predictive Retrosynthesis | Synthesis route design https://www.elsevier.com/products/reaxys/predictive-retrosynthesis (accessed 10/01/2025).
- Latendresse, M.; Malerich, J. P.; Herson, J.; Krummenacker, M.; Szeto, J.; Vu, V.-A.; Collins, N.; Madrid, P. B. Journal of Chemical Information and Modeling 2023, 63, 5484–5495.
- PostEra. PostEra, Medicinal Chemistry Powered by Machine Learning https://app.postera.ai/ (accessed 10/01/2025).
- Molecular AI group. aizynthfinder documentation — aizynthfinder 4.3.1 documentation https://molecularai.github.io/aizynthfinder/ (accessed 10/01/2025).
- Winter, R.; Montanari, F.; Noé, F.; Clevert, D.-A. Chemical Science 2019, 10, 1692–1701.
- Xiong, J.; Zhang, W.; Fu, Z.; Huang, J.; Kong, X.; Wang, Y.; Xiong, Z.; Zheng, M. ChemRxiv 2023.
- Dunn, W. J. Chemometrics and Intelligent Laboratory Systems 1989, 6, 181–190.
- HANSCH, C.; MALONEY, P. P.; FUJITA, T.; MUIR, R. M. Nature 1962, 194, 178–180.
- Kleinstreuer, N.; Hartung, T. Archives of Toxicology 2024, 98, 735–754.
- U.S. Food & Drug Administration. TOXICOLOGY ROADMAP; 2017.
- Center, N. AI Initiatives at NCTR https://www.fda.gov/about-fda/nctr-research-focus- areas/artificial-intelligence (accessed 13/01/2025).
- Chen, X.; Roberts, R.; Liu, Z.; Tong, W. Nature Communications 2023, 14, 7141.
- Li, T.; Chen, X.; Tong, W. npj Digital Medicine 2024, 7.
- Li, T.; Roberts, R.; Liu, Z.; Tong, W. Chemical Research in Toxicology 2023, 36, 916–925.
- Syed Mukhtar Ahmed; Shivnaraine, R. V.; Wu, J. C. Circulation 2023, 148, 309–311.
- Deng, J.; Yang, Z.; Ojima, I.; Samaras, D.; Wang, F. Briefings in Bioinformatics 2021, 23.
- Lavecchia, A. Drug Discovery Today 2015, 20, 318–331.
- Yan, C.; Grabowska, M. E.; Dickson, A. L.; Li, B.; Wen, Z.; Roden, D. M.; Michael Stein, C.; Embí, P. J.; Peterson, J. F.; Feng, Q.; Malin, B. A.; Wei, W.-Q. npj Digital Medicine 2024, 7, 1–6.
- Singh, A. Artificial Intelligence Chemistry 2024, 2, 100071.
- Pesheva, E. Using AI to repurpose existing drugs for treatment of rare diseases https://news.harvard. edu/gazette/story/2024/09/using-ai-to-repurpose-existing-drugs-for-treatment-of-rare-diseases/ (accessed 13/01/2025).
- Rumiana Tenchov; Sasso, J. Rare diseases and orphan diseases: Can combination therapy treat them? https://www.cas.org/resources/cas-insights/rare-diseases-and-orphan- diseases-can-combination-therapy-treat-them (accessed 13/01/2025).
- News-Medical. FB1006: AI-discovered drug advances to clinical trials for ALS treatment https://www.news-medical.net/news/20240227/FB1006-AI-discovered-drug-advances-to-clinical-trials-for-ALS-treatment.aspx (accessed 13/01/2025).
- Garth, E. AI-discovered drug for ALS enters clinical trials https://longevity.technology/ news/ai-discovered-drug-for-als-enters-clinical-trials/ (accessed 13/01/2025).
- Salley, D.; J. Sebastián Manzano; Kitson, P. J.; Cronin, L. ACS central science 2023, 9, 1525–1537.
- Leonov, A. I.; Hammer, A. J. S.; Lach, S.; Mehr, S. H. M.; Caramelli, D.; Angelone, D.; Khan, A.; O’Sullivan, S.; Craven, M.; Wilbraham, L.; Cronin, L. Nature Communications 2024, 15, 1240.
- Rana, A.; Chauhan, R.; Amirreza Mottafegh; Kim, D. P.; Singh, A. K. Communications Chemistry 2024, 7.
- Volk, A. A.; Campbell, Z. S.; Ibrahim, M. Y. S.; Bennett, J. A.; Abolhasani, M. Annual Review of Chemical and Biomolecular Engineering 2022, 13, 45–72.
- Mennen, S. M.; Alhambra, C.; Allen, C. L.; Barberis, M.; Berritt, S.; Brandt, T. A.; Campbell, A. D.; Castañón, J.; Cherney, A. H.; Christensen, M.; Damon, D. B.; Eugenio de Diego, J.; García-Cerrada, S.; García-Losada, P.; Haro, R.; Janey, J.; Leitch, D. C.; Li, L.; Liu, F.; Lobben, P. C. Organic Process Research & Development 2019, 23, 1213–1242.
- Shevlin, M. ACS Medicinal Chemistry Letters 2017, 8, 601–607.
- Chandravadivelu Gopi; Gudapati Krupamai; Chitikina Satya Sri; Magharla Dasaratha Dhanaraju. Beni-Suef University Journal of Basic and Applied Sciences 2020, 9.
- Babak Mahjour; Zhang, R.; Shen, Y.; McGrath, A.; Zhao, R.; Mohamed, O. G.; Lin, Y.; Zhang, Z.; Douthwaite, J. L.; Tripathi, A.; Cernak, T. Nature Communications 2023, 14.
- Domokos, A.; Nagy, B.; Szilágyi, B.; Marosi, G.; Nagy, Z. K. Organic Process Research & Development 2021, 25, 721–739.
- Mascia, S.; Heider, P. L.; Zhang, H.; Lakerveld, R.; Benyahia, B.; Barton, P. I.; Braatz, R. D.; Cooney, C. L.; Evans, J. M. B.; Jamison, T. F.; Jensen, K. F.; Myerson, A. S.; Trout, B. L. Angewandte Chemie International Edition 2013, 52, 12359–12363.
- Coley, C. W.; Thomas, D. A.; Lummiss, J. A. M.; Jaworski, J. N.; Breen, C. P.; Schultz, V.; Hart, T.; Fishman, J. S.; Rogers, L.; Gao, H.; Hicklin, R. W.; Plehiers, P. P.; Byington, J.; Piotti, J. S.; Green, W. H.; Hart, A. J.; Jamison, T. F.; Jensen, K. F. Science 2019, 365, eaax1566.
- Bennett, J. A.; Abolhasani, M. Current Opinion in Chemical Engineering 2022, 36, 100831.
- Milad Abolhasani; Kumacheva, E. Nature Synthesis 2023, 2, 483–492.
- Ekins, S. GEN Biotechnology 2024, 3, 83–86.
- Tom, G.; Schmid, S. P.; Baird, S. G.; Cao, Y.; Darvish, K.; Hao, H.; Lo, S.; Pablo-García, S.; Rajaonson, E. M.; Skreta, M.; Yoshikawa, N.; Corapi, S.; Gun Deniz Akkoc; Strieth-Kalthoff, F.; Seifrid, M.; Alán Aspuru-Guzik. Chemical Reviews 2024, 124, 9633–9732.
- Martin, H. G.; Radivojevic, T.; Zucker, J.; Bouchard, K.; Sustarich, J.; Peisert, S.; Arnold, D.; Hillson, N.; Babnigg, G.; Marti, J. M.; Mungall, C. J.; Beckham, G. T.; Waldburger, L.; Carothers, J.; Sundaram, S.; Agarwal, D.; Simmons, B. A.; Backman, T.; Banerjee, D.; Tanjore, D. Current Opinion in Biotechnology 2023, 79, 102881.
- Rapp, J. T.; Bremer, B. J.; Romero, P. A. Nature Chemical Engineering 2024, 1, 97–107.
- Liverpool, L. Nature 2023, 618, 222–223.
- Van Noorden, R. Nature 2023, 623, 466–467.
- Parker, L.; Boughton, S.; Bero, L.; Byrne, J. A. Journal of Clinical Epidemiology 2024, 176, 111549–111549.
- STM Integrity Hub - STM Association https://stm-assoc.org/what-we-do/strategic-areas/research-integrity/integrity-hub/ (accessed 15/01/2025).
- Proofiger LTD https://www.proofig.com/ (accessed 16/01/2025).
- Robinson, J. Review mills identified as a new form of peer-review fraud https://www.chemistryworld.com/news/review-mills-identified-as-a-new-form-of-peer-review-raud/4018888.article (accessed 16/01/2025).
- Van Noorden, R. Nature 2023, 624, 479–481.
- Supreme people's court of China. Guidelines on Ensuring Technological Innovation with High-Quality Judicial Services https://ipc.court.gov.cn/zh-cn/news/view-3828.html (accessed 16/01/2025).
- Scarfe, P.; Watcham, K.; Clarke, A.; Roesch, E. PloS one 2024, 19, e0305354–e0305354.
- NZQA. https://www2.nzqa.govt.nz/tertiary/assessment-and-moderation-of-standards/academic-integrity-and-artificial-intelligence (accessed 16/01/2025).
- Lee, V. R.; Pope, D.; Miles, S.; Zárate, R. C. Computers and education. Artificial intelligence 2024, 7, 100253–100253.
- White, J. Academic Integrity in the Age of AI https://er.educause.edu/articles/sponsored/2023/11/academic-integrity-in-the-age-of-ai
- Centre for Teaching Excellence University of Waterloo. https://uwaterloo.ca/centre-for-teaching-excellence/Assessment-AI-Strategies (accessed 16/01/2025).
- White, J. https://er.educause.edu/articles/sponsored/2023/11/academic-integrity-in-the-age-of-ai (accessed 16/01/2025).
- Nature 2023, 617, 438–438.
- Nature.com 2024, 636, 273.
- Nobel Prize Outreach AB 2025. Geoffrey Hinton – Banquet speech https://www.nobelprize.org/prizes/physics/2024/hinton/speech/ (accessed 17/01/2025).
- Fitzpatrick, D. Geoffrey Hinton Predicts Human Extinction at the Hands of AI. Here’s How to Stop It. Forbes. December 29 2024