Abstract
The books The Double Helix by John Watson (published in1968) and The Third Man of the Double Helix by Maurice Wilkins (published in 2003), two of the co-discoverers of the structure of DNA in 1953 broke new ground in making public the experiences of practising research scientists: including their personal thoughts, their feelings, and their attitudes to colleagues. This paper uses recent developments in sentiment analysis and in emotional AI (in particular, the latter’s transformer transfer model) to update the previously described trends of sentiment in these books. Visual representation of these trends is demonstrated as complementary to the traditional close reading of such narratives.
Graphical abstract

Introduction
Stories have been an integral part of human culture, serving as a medium for sharing experiences, values, and knowledge across generations. Among the myriad types of narratives, the 'quest' is one of the most enduring and universal. In a quest narrative, the protagonist embarks on a journey to achieve a significant goal, uncover a universal truth, or attain personal transformation. Along the way, the protagonist face various obstacles— which may be physical and psychological—that challenge and shape them. Schemes such as that devised by Christopher Booker delineate the stages of the quest and provide a framework for analysing such narratives (Fig. 1).1
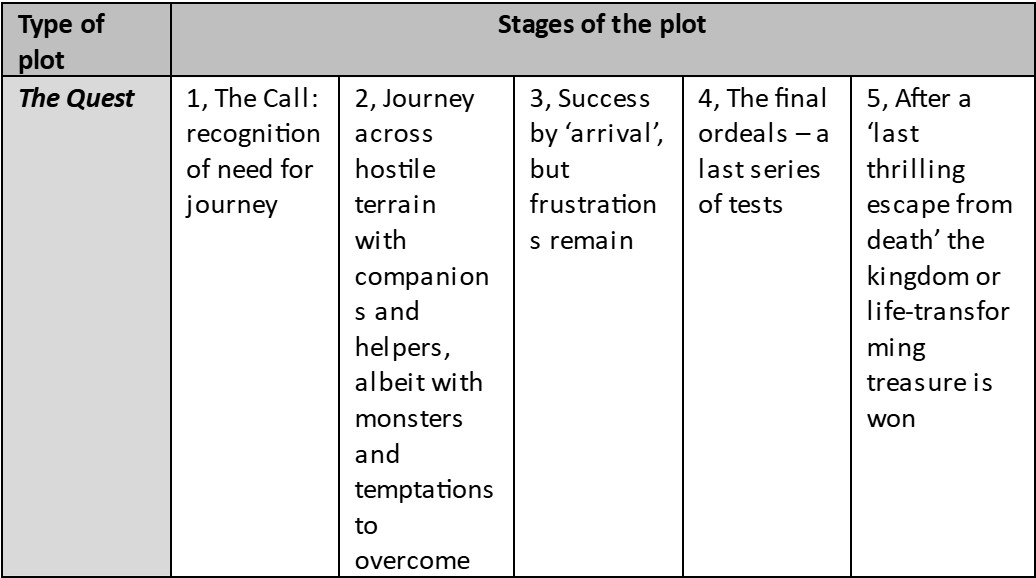
Fig. 1. The Quest: one of seven types of story described by the late Christopher Booker, author of The Seven Basic Plots: Why We Tell Stories1 https://www.theguardian.com/media/2019/jul/04/christopher-booker-obituary
Readers often engage deeply with quest narratives, good examples of which are the quests in each of the books comprising The Lord of the Rings trilogy.2 In this engagement readers connect emotionally with the characters' struggles and triumphs. This emotional engagement is a critical aspect of storytelling that influences how narratives are received and interpreted. Matthew Jockers, a pioneer in computational literary analysis, explored this connection by linking sentiment to the stages of a story (Fig. 2).3 He demonstrated that narratives have inherent emotional arcs that can be quantitatively analysed through sentiment analysis.

The recognition that words (especially adjectives) have emotional overtones is the basis of sentiment analysis. Assignment of positive, neutral, or negative sentiment to a word, determining the frequency of its occurrence in a piece of text, and then repeating it for a large sample of words, enables a calculation of the text’s overall sentiment. As in many ‘training’ applications (see Appendix 1), the larger the sample the more reliable is the sentiment considered to be. Thus, from an initial use of sentiment analysis in assessing the effectiveness of advertising or the opinions expressed on social media, it came to be used for ever longer pieces of text, including fiction and fact-based creative writing. One of the most widely used pieces of software seems to have been that produced by MonkeyLearn,4 which until recently was freely available. Its popularity was probably increased by reliability tests in which it fared better than its market competitors (Appendix 1).
However, traditional sentiment analysis is limited in its capacity to capture the full spectrum of human emotions, generally reducing complex emotional states to being either ‘positive’ or ‘negative’ (or occasionally ‘neutral’). Recent advances in artificial intelligence (AI), particularly in natural language processing models like BERT and RoBERTa (see Appendix 2),5 have enabled more nuanced emotion detection. These models can identify specific emotions such as joy, sadness, fear, anger, surprise, and disgust, providing a richer understanding of the emotional landscape within a text. This paper examines two examples of the use of sentiment analysis and emotion in the story of the discovery of the structure of DNA in 1953, as told by two of the scientists involved. One of these scientists was a postdoctoral research fellow, James. D. Watson (Fig. 3), whose personal experience of the research and his scientist colleagues was chronicled in a best-selling book The Double Helix, first published in 1968.6 The other scientist was Maurice Wilkins (Fig. 4). An older man than Watson, his reminiscences were published as The Third Man of the Double Helix, decades later in 2003 (the year before he died).7


This study addresses two primary research questions:
- 1. How can AI-based sentiment and emotion analysis complement traditional 'close reading'?
- 2. How do the sentiment and emotion profiles of the two books reveal the authors' perspectives on the discovery of DNA's structure?
By exploring these questions, this paper aims to demonstrate that integrating AI techniques with traditional textual analysis provides broader insights into narratives and their emotional trajectories. This interdisciplinary approach can uncover patterns and emotional nuances that may not be immediately apparent through close reading alone, offering a more comprehensive understanding of the texts.
In the following sections, we detail the methodologies employed for sentiment and emotion analysis, present the findings from both books, and discuss how these findings relate to Watson's and Wilkins’ experiences and perspectives. We will also reflect on the implications of combining AI-based analysis with traditional literary methods and consider the potential for future research in this area.
Sentiment analysis of The double Helix
The sentiment of every sentence in his book was determined using the Monkeylearn software package (See Appendix 1),4 and the sentiment percentage and polarity (either ‘positive’ or ‘negative’) for each chapter is displayed in Table 1.8
Table 1. Sentiment, by chapter, in The Double Helix

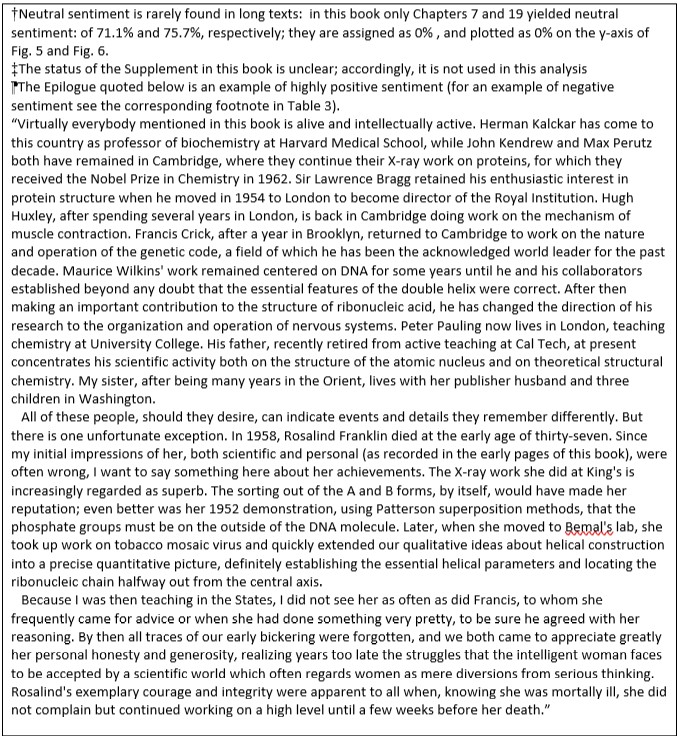
Fig. 5 shows two curves, one in which the amount of text that could be analysed was restricted to short paragraphs(so that the sentiment for a chapter had to be a cumulative or average sentiment); and the other where the complete chapter could be analysed. Both curves show a wide variation of values of sentiment, but by taking 3-chaptermoving averages of sentiment, both broadly similar trends and a variation of sentiment as the story progresses are evident (Fig. 6). In particular, the sentiment is highly positive in the vicinity of Chapter 6, highly negative in the vicinity of Chapter 9, and negative in the vicinity of Chapters 14 and24. Unsurprisingly perhaps, reading of the text of Chapter 6 discloses nothing of particular concern to Watson’s arrangements: neither with his scientific work supervisor Max Perutz nor with his domestic arrangements. In contrast, Chapters 12 and 13 are full of criticism of Watson’s colleague Rosalind Franklin, and Chapters 23 through 25 also include deprecatory statements about her. It would be expected that the ‘close reading’ of text, advocated by humanities scholars, would be consistent with the results of sentiment analysis, and while not exact (caused in part by the averaging process whereby Fig. 6 is generated), there are at least similarities.


On this basis, ‘machine learning’ techniques that have been applied to text and used to compile sentiment scores could be seen as a complement to rather than a replacement of the traditional ‘close reading’ of text that is practised by humanities scholars. Of ‘the close reading, thick description, and deep immersion that erudite scholars can apply to individual works’, philosopher Steven Pinker asks, ‘Must these [three ways] be the only path to understanding?’ Pinker answers his own question with:
‘The first attempts to quantify a concept are always crude, and even the best ones allow probabilistic rather than perfect understanding. Nonetheless quantitative social scientists have laid out criteria for evaluating and improving measurements, and the critical comparison is not whether a measure is perfect but whether it is better than the judgment of an expert, critic, interviewer, clinician, judge, or maven. That turns out to be a low bar.’9
Emotion in The Double Helix
Artificial intelligence offers the opportunity to present a wider range of emotions than the three positive, neutral, or negative categories of sentiment analysis, as described for the ‘transformer transfer learning’ model (see Appendix 2),5 and shown in Fig. 7.

For The Double Helix, each sentence has been analysed for predicted emotions, providing a comprehensive view of emotion throughout the text. For each chapter the emotions in the sentences for the constituent sentences are averaged (Appendix 2, Table 2). The results are plotted in Fig. 8, which shows that the dominant emotion in The Double Helix is joy.
Table 2. AI emotions, by chapter, in The Double Helix*.



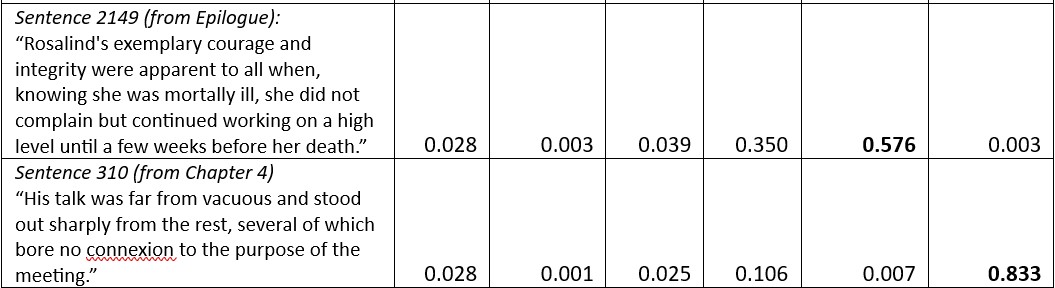

The Double Helix was published in 1968, still only a few years after Watson was awarded a 1/3-share of the Nobel Prize, the most prestigious of 19 scientific prizes and 20 honorary degrees he received after the discovery of the structure of DNA in 1953 (Fig. 9), so his joy in retrospect, especially while writing the preface and the epilogue of his book, is hardly surprising.

Of the other emotions shown in Fig. 8, the emotions ‘surprise’ (which presumably can be both positive and negative in sentiment) and ‘fear’ (inferred to be of negative sentiment) are compared in Fig.10. Fear of being beaten in the race to make a discovery is not uncommon in science, and there is no doubt that in the quest for the structure of DNA, the scientists at King’s College and the Cavendish Laboratory were particularly concerned that the American scientist Linus Pauling would propose the correct structure before they did. The maxima in the dotted blue line of Figure 10 shows clearly that this concern, although there at the start of the project (Chapter 4) was considerably increased towards the end of the quest (Chapter 24).

The trend for ‘sadness’ is shown in Fig. 11. ‘Sadness’ peaks at about half-way through the book, when the relationship of Watson (and others) with Rosalind Franklin (who Watson persistently demeans by calling her ‘Rosy’, while using his other colleagues’ proper names) had deteriorated and was at its most tense. Although peaking at Chapter 15, two examples from Chapter 13 of the text that support this are:
‘Most annoyingly, her objections were not mere perversity: at this stage the embarrassing fact came out that my recollection of the water content of Rosy's DNA samples could not be right. The awkward truth became apparent that the correct DNA model must contain at least ten times more water than was found in our model. This did not mean that we were necessarily wrong. With luck the extra water might be fudged into vacant regions on the periphery of our helix. On the other hand, there was no escaping the conclusion that our argument was soft. As soon as the possibility arose that much more water was involved, the number of potential DNA models alarmingly increased.’ (The Double Helix, p. 80)
and:
‘Our subsequent after-lunch walk into King's and along the backs to Trinity did not, however, reveal any converts. Rosy and Gosling were pugnaciously assertive: their future course of action would be unaffected by their fifty mile excursion into adolescent blather. Maurice [Wilkins] and Willy Seeds [another researcher] gave more indication of being reasonable, but there was no certainty that this was anything more than a reflection of a desire not to agree with Rosy.’ (The Double Helix, p. 80 )
These extracts are associated with the pale blue circle in Fig. 11.
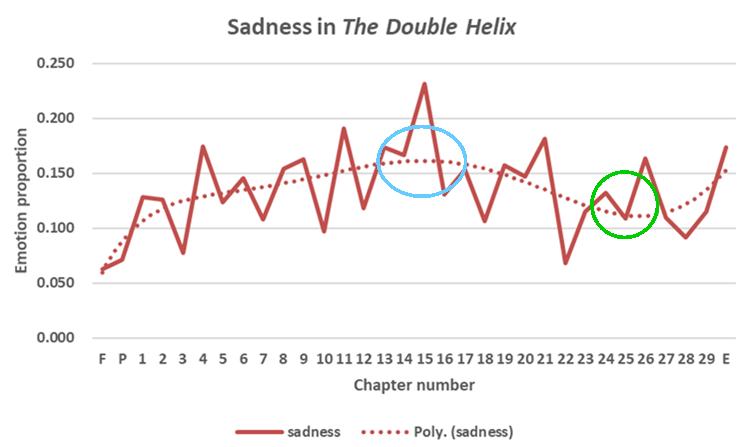
By Chapter 25 (the green circle in Fig. 11), the need for discord between the protagonists was over:
‘In The fact that we had at last produced a stereochemically reasonable configuration for the backbone was always in the back of my head. Moreover, there was no longer any fear that it would be incompatible with the experimental data. By then it had been checked out with Rosy's precise measurements. Rosy, of course, did not directly give us her data. For that matter, no one at King's realized they were in our hands. We came upon them because of Max's membership on a committee appointed by the Medical Research Council to look into the research activities of Randall's lab to coordinate Biophysics research within its laboratories.’
As was the case with sentiment analysis, the inference from the plot of the sadness emotion broadly concurs with that of the ‘close reading’ favoured by humanities scholars. This suggests that artificial intelligence, at least in terms of its application to emotion in writing, can be seen as complementary to – rather than in competition with – other forms of textual analysis. It also enables – as in Figs 8, 10 and 11 a broader view of the full narrative.
Sentiment analysis of The Third Man of the Double Helix
Wilkins’ book comprises ten chapters, each divided into several sections. Sentiment analysis was undertaken for each sentence in the same way as was done for The Double Helix; the sentiment for each section is shown in Table 3.8
Table 3. Sentiment, by chapter section, in The Third Man of the Double Helix
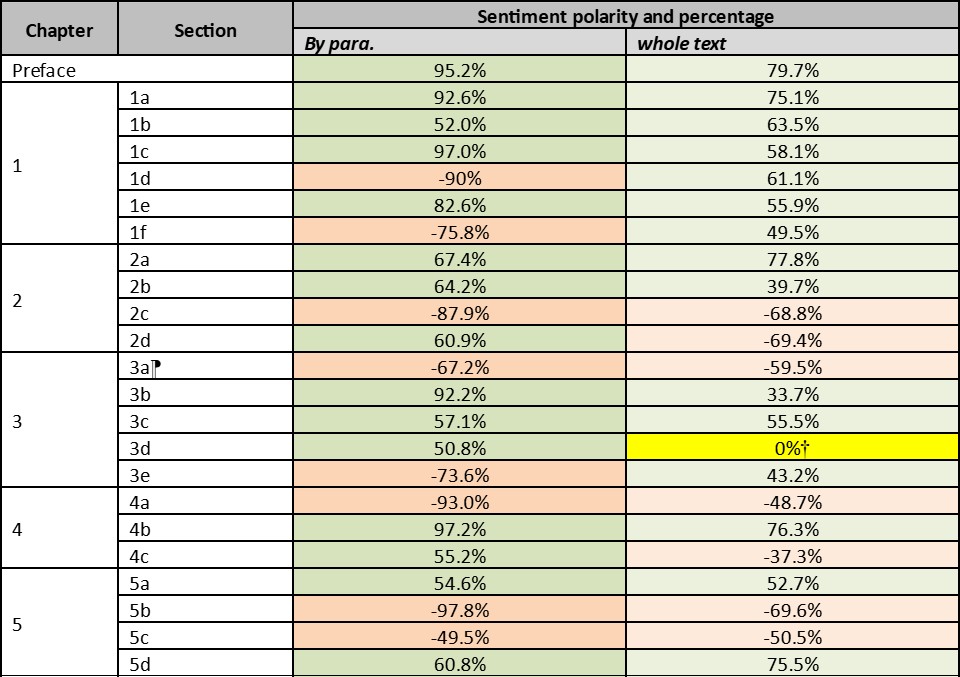

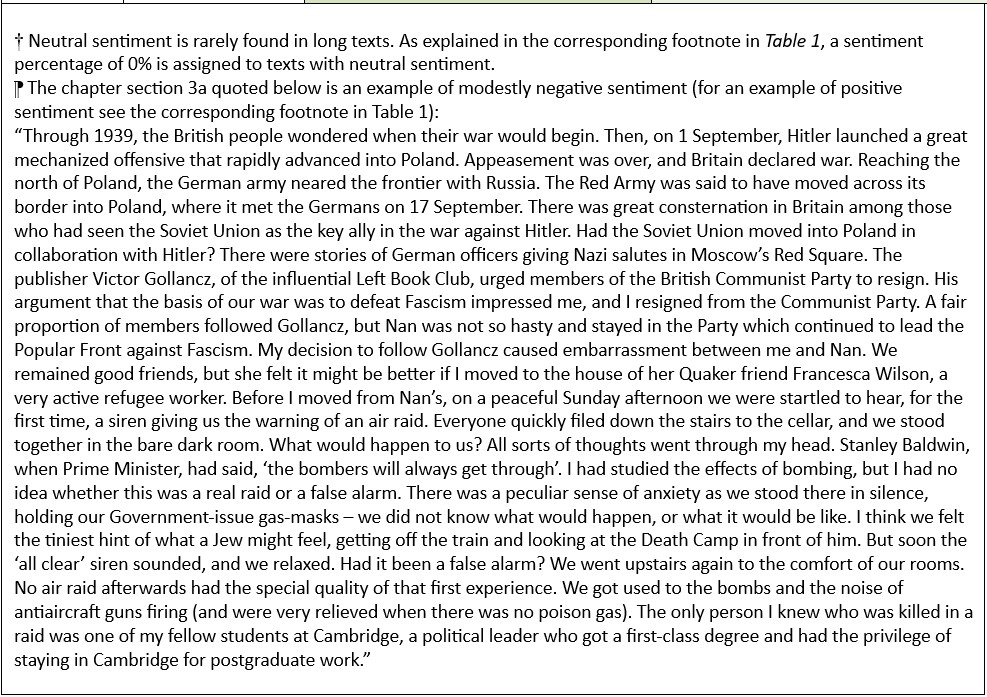
Diagrams corresponding to Fig. 5 and Fig.6 (relating to The Double Helix) are shown as Fig. 12 and Fig.13 (relating to The Third Man of the Double Helix), respectively.


Unlike the plots for The Double Helix (Fig. 5 and Fig. 6), the sentiment plots for the Third Man of the Double Helix (Fig. 12 and Fig. 13) do not resemble that for a quest; rather, the plots are a succession of series of positive maxima and negative minima. Wilkins’ writing is critical of aspects of the research environment, particularly in the earlier and later chapters of the book. Like Watson, Wilkins is critical of Rosalind Franklin, a specialist in X-ray spectroscopy like himself, and this is particularly apparent in later chapters of the book.
Emotion in The Third Man of the Double Helix
The pattern of emotion in Wilkins’ book (see Table 4) differs from that in Watson’s book, undoubtedly caused by the former’s being an autobiography (and thereby descriptive of Wilkins’ whole life), rather than Watson’s emphasis on the DNA project. This is apparent from a comparison of Fig. 8 and Fig 14. Even so, the high proportion of ‘joy’ emotion in both books (albeit lower in Wilkins’ book than Watson’s) is notable, and attests to both scientists’ long-standing personal recognition of the prestige their Nobel-winning research (Fig. 9) brought them.
Table 4. AI emotions, by chapter section, in TheThird Man of the Double Helix



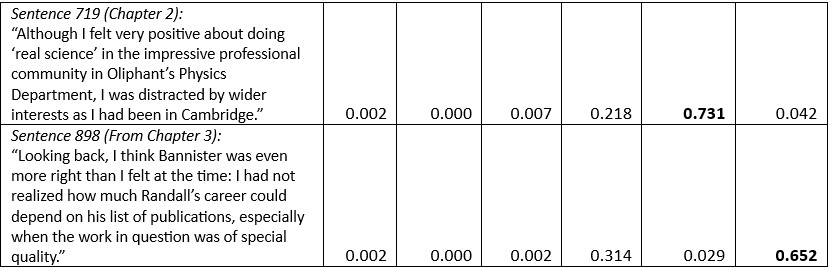
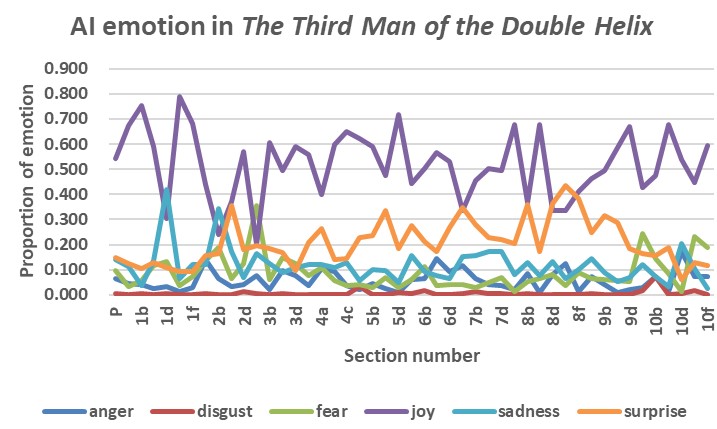
As mentioned in the discussion of The Double Helix, variation in ‘fear’ and ‘surprise’ are expected in scientists’ working experience, as confirmed in Fig. 15. Fear and surprise of the possible turn of World War II cause the peaks in Fig. 15 that corresponded to sections 3a and 2b, respectively. The emotion of ‘surprise’ increasing towards the end of the book, the peaks in Fig. 15 that correspond to sections 8e and 10a relate to Wilkins’ general concerns about limitations that might be imposed on science in the future.

Conclusions
This study aimed to address two primary research questions: “How can AI-based sentiment and emotion analysis complement traditional 'close reading'?” and “How do the sentiment and emotion profiles of the two books, The Double Helix by James D. Watson and The Third Man of the Double Helix by Maurice Wilkins, reveal their authors' perspectives on the discovery of DNA's structure?”
Regarding the first question, our findings demonstrate that AI-based sentiment and emotion analysis can significantly enhance traditional 'close reading' by providing quantitative insights into textual narratives. Traditional close reading involves a detailed, qualitative analysis of a text, focusing on nuances, themes, and stylistic elements. While this method offers deep insights, it can be limited by the subjective interpretations of the reader and may overlook broader emotional patterns within the text.
By applying sentiment analysis tools like MonkeyLearn and advanced AI models such as the Transformer Transfer Learning (TTL) model, we were able to quantify the emotional trajectories within the narratives. For instance, sentiment analysis allowed us to identify peaks and troughs in positive and negative sentiments across different chapters, correlating these fluctuations with specific events and interactions described in the texts. Similarly, emotion detection provided a granular breakdown of emotions such as joy, sadness, fear, anger, surprise, and disgust, offering a multi-dimensional perspective on the authors' emotional expressions.
These quantitative methods complement close reading by highlighting patterns that might not be immediately evident through qualitative analysis alone. They enable scholars to corroborate their interpretations with empirical data, fostering a more holistic understanding of the text. For example, the negative sentiment peaks corresponding to Watson's criticism of Rosalind Franklin were quantitatively validated, reinforcing interpretations derived from close reading.
Addressing the second research question, the sentiment and emotion profiles of the two books offer profound insights into the authors' personal experiences and perspectives on the DNA discovery. In The Double Helix, sentiment analysis revealed significant fluctuations, with notable negative sentiment associated with chapters discussing tensions with Rosalind Franklin and fears of being outpaced by competitor Linus Pauling. The dominant emotion throughout Watson's narrative was 'joy,' particularly heightened in the preface and epilogue, reflecting his retrospective pride and satisfaction with the monumental achievement. The presence of 'fear' and 'sadness' at specific points aligns with the competitive and sometimes contentious atmosphere of the scientific pursuit.
In contrast, The Third Man of the Double Helix presented a more balanced emotional landscape. While 'joy' remained the dominant emotion, indicative of Wilkins' acknowledgment of the significance of their discovery, there were consistent undercurrents of 'fear' and 'sadness.' These emotions peaked in sections discussing World War II's impact and the strained relationships within the research team, including his interactions with Franklin. Sentiment analysis showed less extreme fluctuations compared to Watson's account, suggesting Wilkins' more reflective and measured perspective on the events.
The comparative analysis highlights how the authors' individual experiences, positions, and retrospective reflections shaped their narratives. Watson's account is imbued with the enthusiasm and urgency of a young scientist deeply immersed in a competitive race, whereas Wilkins offers a more nuanced and contemplative recollection, possibly influenced by his seniority and the passage of time before writing his memoir.
In conclusion, the integration of AI-based sentiment and emotion analysis with traditional close reading provides a robust framework for textual analysis. It allows scholars to validate and enrich their qualitative interpretations with quantitative data, uncovering deeper layers of meaning within narratives. Specifically, in the context of these two seminal works on the discovery of DNA's structure, the combined approach elucidates how personal perspectives and emotional undertones influence the portrayal of historical scientific events.
This study underscores the potential for interdisciplinary methodologies to enhance our understanding of literature and historical accounts. By bridging computational analysis with humanistic inquiry, researchers can achieve a more comprehensive and nuanced interpretation of texts. Future research could extend this approach to a broader range of narratives, explore the integration of additional AI models for sentiment and emotion detection, or examine the impact of these emotional trajectories on readers' engagement and interpretation. The findings also invite reflection on the ethical considerations of using AI in literary analysis, such as the importance of contextual understanding and the limitations of algorithms in capturing the full spectrum of human emotion and intent. As AI technologies continue to evolve, their application in the humanities offers promising avenues for innovation while emphasising the enduring value of human insight in interpreting complex narratives.
Appendix 1: Reliability of Monkeylearn sentiment analysis software
Despite a reservation that applying Monkeylearn to tweets – which, being short texts may be unrepresentative,10 in an example of tweets related to a particular earthquake, researchers “obtained an overall accuracy of 63% and a misclassification rate of 37% [which they considered] is sufficient for a preliminary assessment”.11 Using data from Twitter and Facebook the MonkeyLearn tool4 has also been demonstrated as preferable to three other programmes (viz., Sentiment Analyzer, Aylien, and ParallelDots) in terms of its accuracy and precision.12 Also reassuring is the good agreement between sentiment analysis of health reviews undertaken by humans compared with that undertaken by three automatic sentiment analysis tools.13 Of the three tools compared (MonkeyLearn, ACDAPI [Amazon Comprehend Detect Sentiment API], and Google), Monkeylearn correlated the best with the analysis undertaken by human beings. Moreover, they noted that “in this study only off the shelf solutions were assessed, this was due to the balance of cost and time pressures against overall accuracy”,13 from which it is inferred that they may have used the tool in a similar way to that used in this paper.
Appendix 2: The ‘transformer transfer learning’ model
To analyse the emotions present in the texts, we utilised advanced artificial intelligence tools based on transformer models, specifically the Transformer Transfer Learning (TTL) approach.14 Transformer models like BERT (Bidirectional Encoder Representations from Transformers)15 and its derivatives (e.g. RoBERTa)16 represent a significant advancement in natural language processing. They are designed to understand the context and nuances of language by examining the relationships between words in a sentence.
Unlike traditional sentiment analysis, which categorises text into broad sentiments like positive, neutral, or negative, transformer-based models can identify specific emotions such as joy, sadness, anger, surprise, and others. They achieve this through a mechanism called "self-attention," which allows the model to weigh the importance of each word relative to others in a sentence, effectively capturing subtle emotional cues.
The TTL model is a specialised application of RoBERTa through transfer learning, meaning it has been adapted from a general language understanding model to a specific task, in this case, emotion detection. This model has been fine-tuned on extensive emotion-labeled datasets: over 3.6 million sentences from four self-reported emotion datasets and more than 60,000 sentences from seven annotator-rated datasets. This fine-tuning enables the TTL model to map emotions expressed in text comprehensively, achieving an impressive accuracy rate of up to 84% in emotion detection.
By providing probability scores for each identified emotion in a sentence, tools like the TTL model allow for a granular understanding of the emotional states conveyed in the text. In our study, this approach enabled us to detect and quantify emotions such as joy, sadness, fear, anger, and surprise within the narratives of The Double Helix and The Third Man of the Double Helix. This level of detail goes beyond what traditional sentiment analysis can offer, allowing for a deeper exploration of the emotional trajectories and nuances in the book-authors' accounts.
References
- Booker, C. The Seven Basic Plots: Why We Tell Stories. Continuum: London, 2004, 9-86.
- Tolkien, J.R.R. The Fellowship of the Ring, The Two Towers, The Return of the King. Allen and Unwin: London, 1954, 1954, 1955.
- Jockers, M. A novel method for detecting plot, 2014. https://www.matthewjockers.net/2014/06/05/a-novel-method-for-detecting-plot/ ; Jockers, M. Revealing sentiment and plot arcs with the Syuzhet package, 2015. https://www.matthewjockers.net/2015/02/02/syuzhet/
- Sentiment analyzer. Monkeylearn, undated. https://monkeylearn.com/sentiment-analysis-online/
- Lee, S.J.; Ahn, H.S.; Paas, L. The Conversation 2024 (October 1). https://theconversation.com/happy-sad-or-angry-ai-can-detect-emotions-in-text-according-to-new-research-239376
- Watson, J.D. The Double Helix: A Personal Account of the Discovery of the Structure of DNA. Penguin: London, 1968.
- Wilkins, M. The Third Man of the Double Helix: An Autobiography of Maurice Wilkins. Oxford University Press, 2003.
- Hodder, P. The double helix revisited. Chemistry in New Zealand (online), 2024. https://www.cinz.nz/posts/%20the-double-helix-revisited
- Pinker, S. Enlightenment Now. Penguin Randon House: UK, 2018, p. 408.
- Yazman, J. How much text do we really need for sentiment analysis? 2017. https://rpubs.com/joshyazman/sample-size-needed-for-text-analysis
- Contreras, D.; Wilkinson, S.; Alterman, E.; Hervás, J. Natural Hazards 2022, 113 (1), 403–421. doi.org/10.1007%2Fs11069-022-05307-w
- Md Nor Basmmi, A.B.; Shaliza, A.H.; Nor, S. IOP Conference Series Materials Science and Engineering 2020, 884 (1), paper 012063. http://dx.doi.org/10.1088/1757-899X/884/1/012063
- Byme, M.; O’Malley, L.; Glenny, A.-M.; Pretty, I.; Tickle, M. PLOS One 2021, 16 (12), paper e0259797. https://doi.org/10.1371/jounal.pone.0259787
- Lee, S. J.; Lim, J.; Paas, L.; Ahn, H.S. Neural Computing and Applications 2023, 35(15), 10945-10956.
- Devlin, J.; Chang, M.W.; Lee, K.; Toutanova, K. arXiv preprint arXiv 2018, 1810.04805,15. https://doi.org/10.48550/arXiv.1810.04805
- Liu, Y.; Ott, M.; Goyal, N.; Du, J.; Joshi, M.; Chen, D. et al. arXiv preprint arXiv 2019, 1907.11692, 364. https://doi.org/10.48550/arXiv.1907.11692